In the pursuit of providing on-demand and in-the-moment insights to consumers, we have come a long way. Our applications are high-performing, scalable, and more responsive. This happened because we embraced automation and built smarter analytics solutions. That too, without compromising upon the ethos of confidentiality. However, amidst all this, we missed our crucial part – QA. Despite the Test Data Management market pacing to a CAGR growth of 12.7% till 2022 end, test teams are locking horns with challenges and often end up making critical mistakes.
Here’s more:
Unplanned Data Collection from a Multitude of sources
Data is often scattered across various internal and external databases. This is primarily why data extraction consumes a lot of time. Since testing teams have limited access to production and backup systems, they are unable to deal with a large number of schemas and databases.
This only entails that such organizations are dependent on their stakeholders like database admins and subject matter experts to give them the data they need. This mounts up to the excessive loss of time during the testing process.
One such approach is offered by K2view for this problem. The data fabric doesn’t store data based on type. Rather, it stores all data types for a particular business entity in an exclusive micro-database. Such a data schema is the only point of source to query any data related to the business entity.
While we are at it, a business entity holds all data types such as customer name, address, credit card number, social security number, and others. With such an approach, the data is queried for business needs in real-time without any pre-defined structuring.
How does it help TDM? The ecosystem pulls data from multiple sources, accumulates them into business entities, and creates synthetic test data. This ensures seamless provisioning for any type of downstream environment.
Not Masking Sensitive Data
Over the years, the rising concerns around data security would result in total spending of USD 170 bn by the end of 2023. As the consumer adoption of digital services such as money wallets, social platforms and others increases, the risk of theft will always be there.
There’s an unimaginable volume of personal data across systems such as social security numbers, credit cards, mobile numbers, bank accounts, purchasing details, etc. While business applications have multiple layers to protect consumer data (lying at product systems) confidentiality, testing environments continue to be at a greater risk.
As soon as the production data is exposed to unsecured 3rd party testing environments, it becomes an easy target for hacks and infiltration attempts. Since your QA partner could be anywhere in the world, they may or may not be working in compliance with customer confidentiality guidelines that your jurisdiction follows. At some times, they may not even consider the sensitivity of data in their scope.
Centralized Test Data Management Approach
Web 3.0 is impacting enterprise processes across the system landscape. It is totally in contrast to the centralized storage that pillared web 2.0. TDM too should undergo this makeover. Here’s why.
In many organizations, the ownership of test data functions stays with a centralized team that is independent of DevOps & Agile Sprint teams. Since there’s a large volume of test data sets required in real-time, the centralized team is often entangled in lengthy provision cycles.
As a result, the dependent teams (DevOps & Agile) are unable to benefit from continuous testing sessions. This is just one of the issues with centralized processing. Others include extracting data from multiple sources and providing it to different environments slows the practice and increases cost.
As discussed above, decentralized storage would fasten the process wherein every node would provide the needed data set to the test environment. All of this without breaching the standards set for the entire system.
Practicing Poor Data Profiling
Developing high-performing data integration applications needs accurate data profiling. For ETL developers, it helps to clean and process data sets. However, poor data profiling can disrupt the TDM practice in the long run.
For example, customer ‘A’ is found with the country code in the ‘phone_number’ field. At this point, an ETL instruction to extract the ‘country_code’ data from the ‘phone_number’ field and move to ‘country_code’ will work. What if there are more customer entries with the same issue? Writing exact instructions for every entry isn’t optimal.
Such complexities arise when in-depth data profiling is ignored. TDM teams should consider the unpredictable nature of the data sets. What’s working for an existing data set may not work for all upcoming sets in the future. Therefore, it is imperative to perform data profiling at the beginning so that minimum effort goes into cleaning and updating the sets.
Conclusion
So far, we have discussed common mistakes at the strategic level that put a TDM process at unimaginable risk. While the challenges of TDM are inevitable, enterprises must embrace contemporary practices and prepare for web 3.0. It’s the beginning and the right time to make the move. What complexities are you facing at TDM? Do share.
I am an Entrepreneur who co-founded Mobodexter and Hurify groups, a technology disrupter, influencer and board member on Blockchain & Smart City projects worldwide.
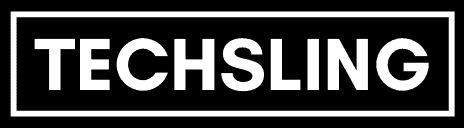